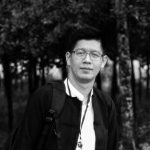
The author’s interest in investigating the suicide problem in Thailand during the specified time stems from the increasing recognition of suicide as a significant public health issue. Thailand, like many other countries, faces complex social and economic challenges that may influence mental health and suicide rates. By examining the determinants of suicide rates across different provinces, the author aimed to identify specific risk factors and their spatial distribution, thereby providing a deeper understanding of the underlying causes. This research is crucial for informing targeted public health interventions and policies to mitigate suicide risks and improve overall mental health outcomes in Thailand.
One of the potential risk factors mentioned in the paper is household income. Interestingly, the study found that higher household income is associated with increased suicide rates in Thailand. This counterintuitive finding suggests that economic affluence might contribute to social isolation, a factor that can significantly impact mental health. Higher-income individuals may experience greater pressure to maintain their economic status, leading to stress and anxiety. Additionally, wealthier individuals might have weaker community ties compared to those in lower-income brackets, where social cohesion is often stronger due to shared economic struggles. This lack of strong social support networks can leave individuals more vulnerable to mental health issues and suicidal behaviors. Therefore, understanding the role of income as a risk factor highlights the need for mental health support services that are accessible to all economic groups and the importance of fostering community connections regardless of economic status.
Statistical modeling plays a vital role in investigating the epidemiology and spatial aspects of the suicide problem in Thailand. By applying advanced statistical techniques, researchers can analyze complex data sets to identify significant risk factors and their interactions. Models allow for the control of confounding variables and the assessment of potential effect modifiers, providing a clearer picture of the relationships between different determinants and suicide rates. Spatial epidemiology, in particular, enables the examination of geographical patterns and clustering of suicides, highlighting areas with higher risks and facilitating targeted interventions. Bayesian models and spatio-temporal analyses can incorporate prior knowledge and account for spatial dependencies, improving the accuracy and reliability of the findings.