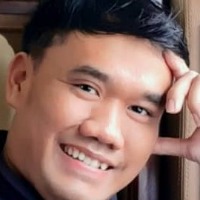
Big data in cardiovascular disease (CVD) is leverage to improve treatment, research, and development of CVD. However, management of big data contains significant issues where capacity of human resources (health informaticians or data scientists) is the key to addressing any significant complexities. Here are some practices of coping mechanisms when dealing with challenges of big health data:
a. Handling any missing data: although this issue can be overcome by using statistical approaches (imputation, regression, estimation, and inference), understanding how to select those methods must be done carefully to mitigate the high number of missing values.
b. Avoiding selection bias: it is about how researchers defend their principles when are intervened by data and science. Avoiding selection bias is a difficult challenge, but it is true that large volumes of big data do not always imply a representative sample with any valid inference. The electronic health record of CVD patients is likely to store exposure history and risk factor information that is scientifically related to CVD, but these cannot be generalized for decision-making. In clinical research, randomized controlled trials have the greatest influence.
c. Mainstreaming ethical clearance and data security (protecting the subject’s personal information from malicious actions): implement internet and medical data encryption, multilayer authentication, firewalls, and administrative safeguards under the principle of data confidentiality-integrity-availability.
d. Integrating big data management into healthcare workforce education: apart from becoming a challenge, big data is actually an opportunity for higher education institutions to prepare digital-literate health workers. Education and training curricula must contain big data applications so that every health worker can be ready to actualize clinical service in line with the nowadays demands.
e. Interpreting the correct translation: huge volume, variety, and velocity of big CVD data enables multiple assumptions. Once the users play with big data, they should be able to execute visualization and text mining in order to provide the appropriate translation.