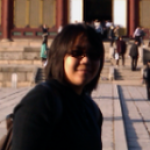
According to “BIG HEALTH DATA CHALLENGES” in this paper, there are different types of challenges facing the implementation. For the possible method of handling these issue as below:
1) Missing Data: This is one of the most common problems which can reduce the statistical power of a study and can produce biased estimates, leading to invalid conclusions. To prevent the problem by well-planning the study and collecting the data carefully. The following are suggested to minimize the amount of missing data in clinical research:
1.1) Should limit the collection of data to those who are participating in the study, collecting only the essential information at each visit.
1.2) Before the beginning of the clinical research, a detailed documentation of the study should be developed in the form of the manual of operations, which includes the methods to screen the participants, methods to communicate between the investigators or between the investigators and participants, implementation of the treatment, and procedure to collect, enter, and edit data.
1.3) Before the start of the participant enrollment, training should be conducted to instruct all personnel related to the study on all aspects of the study.
1.4) Should set priority targets for the unacceptable level of missing data. With these targets in mind, the data collection at each site should be monitored and reported in as close to real-time as possible during the course of the study.
1.5) If a patient decides to withdraw from the follow-up, the reasons for the withdrawal should be recorded for the subsequent analysis in the interpretation of the results.
2) Selection Bisa: It is a kind of error that occurs when the researcher decides who is going to be studied. It is usually associated with research where the selection of participants isn’t random.
2.1 Try to make a study representative by including as many people as possible.
2.2. Using random methods when selecting subgroups from populations.
2.3 Ensuring that the subgroups selected are equivalent to the population at large in terms of their key characteristics.
3) Data Analysis and Training:
3.1 Prepare dataset what suitable for study.
3.2 Train to upskill and knowledge for the researcher, for example, data science, statistics, program usage, database management etc.
4) Interpretation and Translational Applicability of Results:
4.1 Prepare the adequate description of the dataset variables and associated metadata.
4.2 Prepare and control the quality of data which use in research.
5) Privacy and Ethical Issue:
5.1 For personal data, it must be collected in a lawful and fairway for a purpose directly related to a function/activity of the data user.
5.2 Information on a patient should be released to others only with the patient’s permission or allowed by law.
5.3. Information shared as a result of clinical interaction is considered confidential and must be protected.
5.4 To train research staff about privacy and confidentiality issues that may arise when collecting data. The research staff should not open drawers or closets without permission and should collect samples only in pre-approved, designated areas.