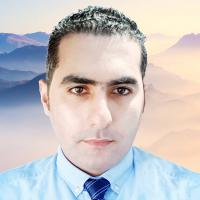
In my opinion, We can implement several strategies to face these challenges including:
Disease definition: The quality and comparability of big health data can be enhanced by developing standardized and consistent disease definitions.
Data availability: Big health data can be made more accessible by forming alliances with a variety of data sources by developing partnerships to increase data availability with various data sources, such as hospitals, clinics, and research institutions. Also by involving cloud-based solutions and platforms to store and share large amounts of data, making it more accessible for remote teams and researchers.
Data quality and missing data: Data quality can be improved and missing data can be addressed using methods like data validation to check the data for any errors or inconsistencies, imputation by using statistical techniques to fill in missing data based on the observed data, and data cleaning to identify and remove errors, outliers, and inconsistencies in the data.
Data inconsistency and Data integrity: Data inconsistency can be addressed by developing methods for integrating and standardizing data from multiple sources. The integrity of large amounts of health data can be safeguarded by putting in place stringent data security and privacy measures.
Training: Developing specialized training programs for individuals working with big health data can help to ensure that they have the necessary skills and knowledge to work with this type of data.
Legal and ethical issues: Developing policies and guidelines to address legal and ethical issues related to big health data can help to mitigate risks and ensure compliance. And improve healthcare organizations’ transparency about how they collect, use, and share health data.
Data security: Implementing robust security measures such as encryption, firewalls, and network segmentation can help to protect big health data from unauthorized access and breaches. Data can be encrypted to protect it from unauthorized access or breaches.
Fairness and bias: Implementing fairness-aware machine learning methods and monitoring the data and models for any potential bias can help to mitigate any unfairness in the predictions.
Overall, it’s important to combine technical solutions such as encryption, access controls, network security, and data backup with policy, and governance, and collaborate with domain experts and stakeholders to ensure that the data and models are fair and align with ethical and social values.